Exploring Maximum Flow Problems: The Ford-Fulkerson Algorithm
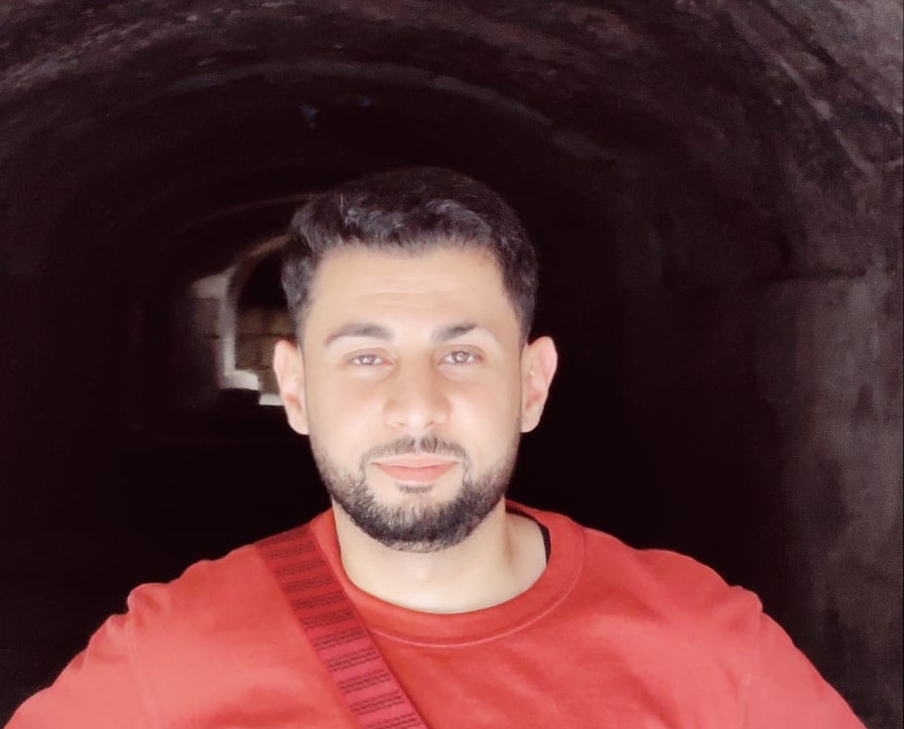
MontaF - Nov. 16, 2024
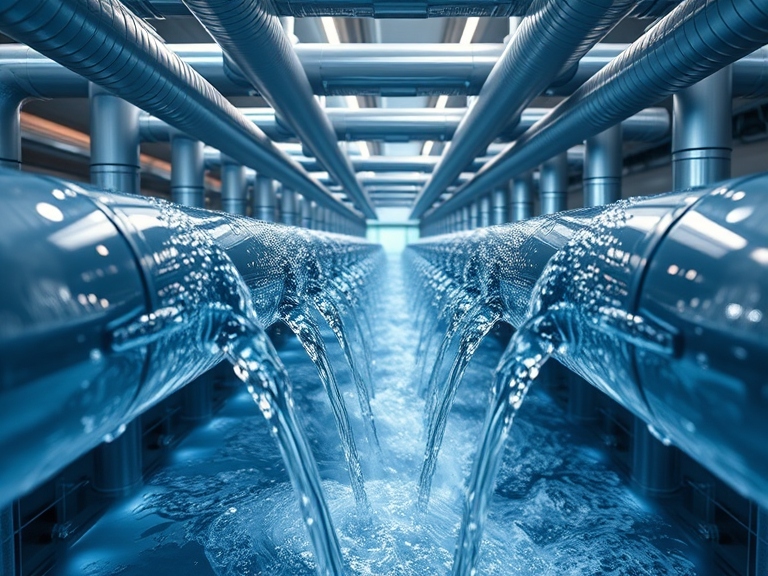
When solving real-world problems involving networks — whether it’s optimizing transportation systems, routing data efficiently, or even creating matchmaking algorithms — the concept of maximum flow plays a pivotal role. In this post, we’ll explore the Ford-Fulkerson Algorithm, complete with Python code snippets, to make the learning process insightful.
Understanding the Maximum Flow Problem
What is Maximum Flow?
Imagine a network of pipes where water flows from a source S
to a sink T
. Each pipe has a limit on how much water it can carry, known as its capacity. The goal is to determine the maximum possible flow from S
to T
without exceeding these capacities.
This scenario translates into graph terms:
- A directed graph
G(V,E)
, whereV
is the set of vertices, andE
is the set of edges. - Each edge
(u,v)
has a capacityc(u,v)
. - You need to calculate the maximum flow from
S
toT
under the capacity constraints.
Applications
Some real-world applications of maximum flow problems include:
- Data Networks: Optimizing bandwidth usage.
- Transportation: Managing traffic or logistics.
- Matching: Assigning tasks to workers efficiently.
- Infrastructure: Balancing load across utility networks.
The Ford-Fulkerson Algorithm
The Ford-Fulkerson Algorithm is a powerful technique to solve maximum flow problems. Its core idea is simple:
- Find a path from
S
toT
where additional flow is possible (called an augmenting path). - Push as much flow as possible along this path.
- Repeat until no more augmenting paths exist.
Residual Graph
To keep track of the available capacities, the algorithm uses a residual graph. If f(u,v)
is the current flow and c(u,v)
is the capacity, the residual capacity r(u,v)
is:
r(u,v)=c(u,v)−f(u,v)
Let’s break it down step by step.
Step-by-Step Explanation with an Example
Graph Representation
Here’s a network example. The capacities of edges are labeled.
Python Implementation
First, let’s represent the graph in Python using adjacency lists.
from collections import defaultdict class Graph: def __init__(self, vertices): self.graph = defaultdict(list) # Adjacency list self.capacity = {} # Edge capacities self.V = vertices # Number of vertices def add_edge(self, u, v, capacity): self.graph[u].append(v) self.graph[v].append(u) # Add reverse edge for residual graph self.capacity[(u, v)] = capacity self.capacity[(v, u)] = 0 # Reverse edge starts with 0 capacity
Step 1: Finding Augmenting Paths
We use a Breadth-First Search (BFS
) to find a path from S
to T
in the residual graph.
def bfs(self, source, sink, parent): visited = [False] * self.V queue = [source] visited[source] = True while queue: u = queue.pop(0) for v in self.graph[u]: # If there's available capacity and v is not visited if not visited[v] and self.capacity[(u, v)] > 0: queue.append(v) visited[v] = True parent[v] = u # Store the path if v == sink: return True return False
Step 2: Augmenting Flow
For every augmenting path found, calculate the bottleneck capacity (minimum capacity in the path) and update the flows and residual capacities.
def ford_fulkerson(self, source, sink): parent = [-1] * self.V # To store the path max_flow = 0 while self.bfs(source, sink, parent): # Find the bottleneck capacity path_flow = float('Inf') s = sink while s != source: path_flow = min(path_flow, self.capacity[(parent[s], s)]) s = parent[s] # Update residual capacities v = sink while v != source: u = parent[v] self.capacity[(u, v)] -= path_flow self.capacity[(v, u)] += path_flow v = parent[v] # Add path flow to overall flow max_flow += path_flow return max_flow
Walkthrough Example
Let’s test the implementation with our example graph:
g = Graph(6) g.add_edge(0, 1, 16) g.add_edge(0, 2, 13) g.add_edge(1, 2, 10) g.add_edge(1, 3, 12) g.add_edge(2, 1, 4) g.add_edge(2, 4, 14) g.add_edge(3, 2, 9) g.add_edge(3, 5, 20) g.add_edge(4, 3, 7) g.add_edge(4, 5, 4) source = 0 sink = 5 print("The maximum possible flow is:", g.ford_fulkerson(source, sink))
Output:
The maximum possible flow is: 23
Algorithm Complexity
Time Complexity: O(E × max_flow)
, where E
is the number of edges and max_flow
is the value of the maximum flow. This can be improved using the Edmonds-Karp Algorithm, which limits the search with BFS for O(VE2)
.
Real-World Applications
- Internet Traffic Optimization: Balancing data across servers.
- Task Assignment: Matching workers to jobs efficiently.
- Transportation: Optimizing flow of goods or traffic.
- Network Design: Ensuring reliability and redundancy.
Conclusion
The Ford-Fulkerson Algorithm is an elegant and intuitive approach to solving maximum flow problems. Its simplicity and adaptability make it a foundational tool in computer science and operations research. Whether you're optimizing a network or designing a system, mastering this algorithm gives you an edge in tackling a wide range of challenges.
Want to explore other algorithms like Edmonds-Karp or Dinic’s? Let’s discuss in the comments!